Network security is regarded as one of the main concerns for the internet of things. As 5G rolls out and 6G advances, new threats and attacks opportunities arise – including for vehicles using federated learning.
A recent experiment has indicated that Simulated Poisoning and Inversion Network (SPIN) in federated learning can reduce the machine learning model’s accuracy by 22% using one single attacker. That means autonomous cars would send and receive the wrong models as they travel, putting drivers and passengers at risk.
In a traditional ML environment, the training data is uploaded from the user’s device to a centralised cloud space. Then the system analyses the dataset and learns from it, creating a model.
In federated learning, on the other hand, the data stays in the user’s equipment – a smartphone or a car, for example – and the ML part of the process happens in the device. In this case, the model is sent to the cloud, not the data itself, making it more difficult for hackers to access sensitive information.
The experiment, however, shows that even federated learning can be subject to attacks. It was carried out by researchers at the University of Sindh (Pakistan), Munster Technological University (Ireland), and Nearby Computing (Spain).
“The emergence of model inversion attacks that can reproduce the data from model weights (gradient maps) render federated learning methods vulnerable to security threats,” the authors say in an article summarising the results.
Attacking the System
In the experiment, the researchers gathered models from ten cars – one of them being the attacker. According to them, the SPIN framework consisted of three steps:
- Using a differential model for generating the data from model weights (gradients);
- The generated data underwent adversarial attacks, followed by a generative adversarial network that created ‘poisoned’ – that is, inaccurate or bogus – data, and
- A local model using degraded data was trained and sent to the roadside Unit [RSU] to be aggregated into the global model.
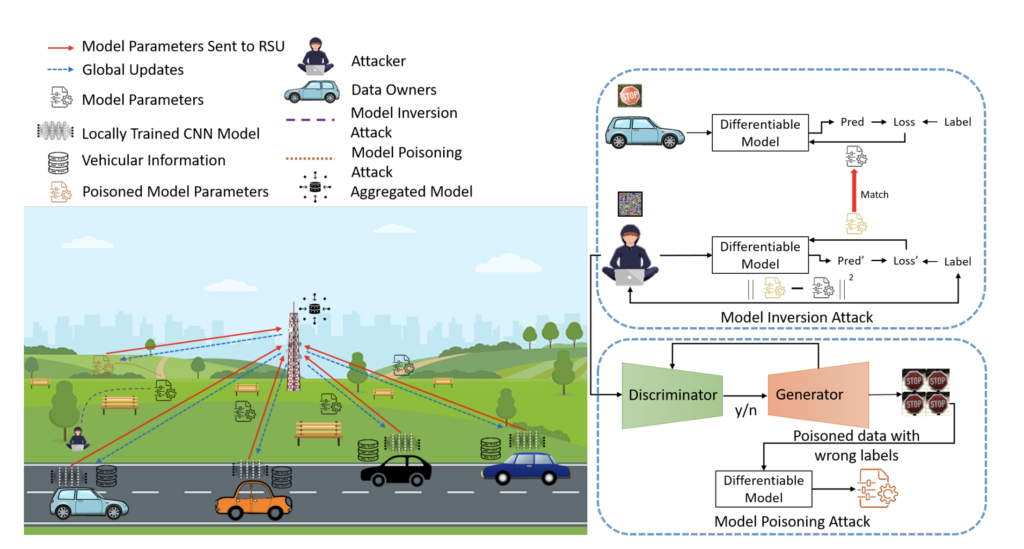
The researchers used two datasets – MNIST (a handwritten digit database) and GTSRB (German Traffic Sign Benchmark).
In both cases, the accuracy of the global model drastically dropped over time.
As the model was corrupted back and forth in every round of communication with the roadside unit (illustrated by the antenna in the picture), accuracy plunged by 22%.
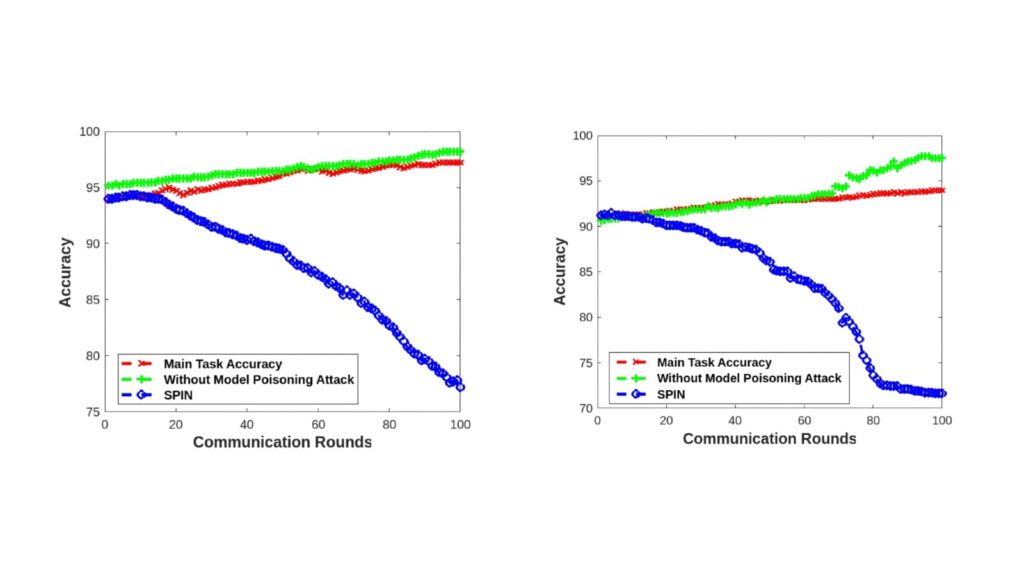
“The proposed work has illustrated the efficacy of attacks by mimicking the data from the model and then poisoning the labels with respect to the federated learning process. It is a kind of a sneak attack that camouflages itself as one of the participants by reconstructing the data from the differential model rather than invading other participant’s data for generating poison attack,” the authors said.
“As future work, we plan to conduct more experiments with a varying number of attackers and benign vehicles to validate the efficacy of SPIN architecture. Furthermore, we also intend to propose a defense mechanism for such attacks concerning federated learning-based vehicular applications,” they added.